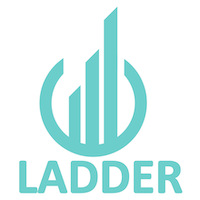
LADDER, an acronym for Labeling and Detection Deployment for Entity Recognition, has transformed the dream of creating customized computer vision systems into reality, making it accessible to many without the need for programming skills. Utilizing a laptop and dedicating just a few hours, users can leverage LADDER's Graphic User Interface (GUI) to perform object labeling, neural network training, and object detection in images—all without writing a single line of code. For instance, a system capable of counting seeds can be easily developed by simply labeling a few wheat kernels. This system is not only effective for wheat but is also adaptable to other grains such as beans. The ability to label objects on detected images streamlines the process of gathering more training data, which in turn enhances the system's accuracy. By training the system on images of fragmented and whole rice separately, users can quickly establish a basic yet effective system. This is particularly useful for evaluating the ratio of whole rice, a vital factor in determining rice price. Moreover, LADDER's capabilities extend to assessing the severity of stripe rust at the resolution of individual rows, showcasing its versatility and efficacy in various applications.