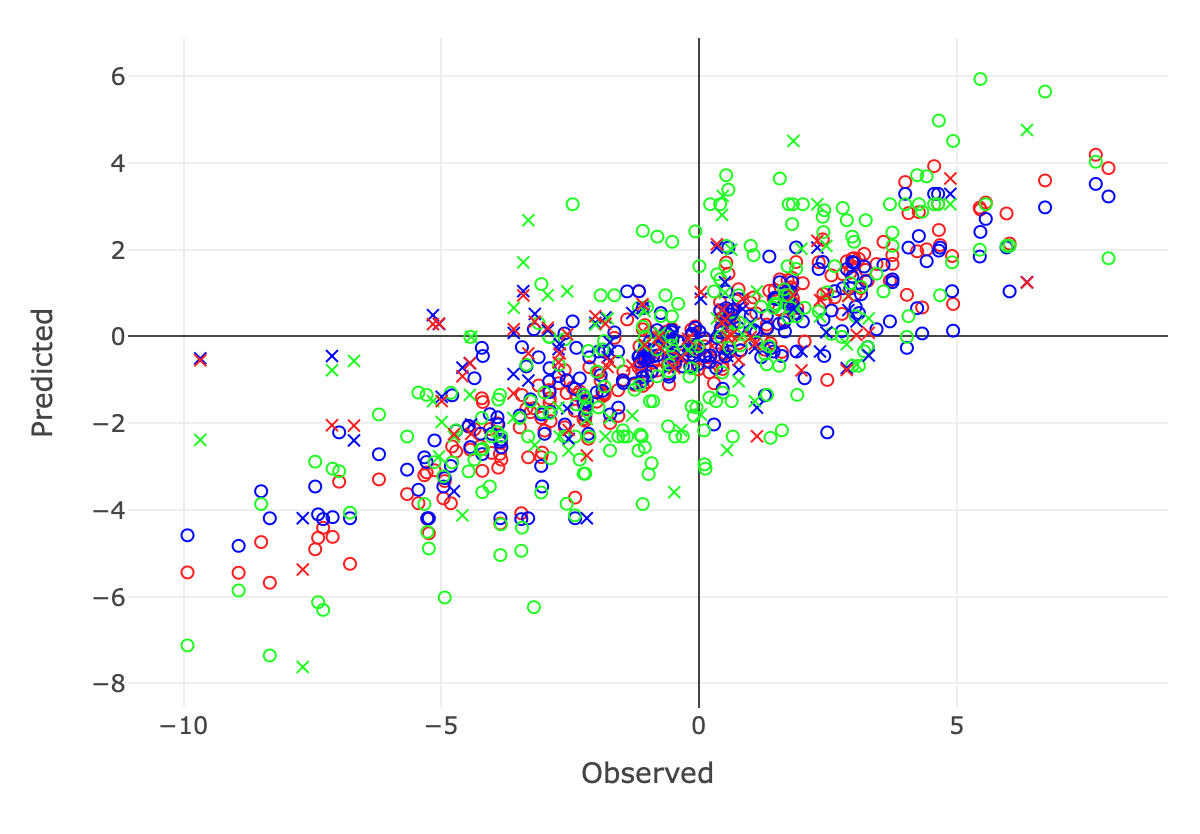
Genomic Prediction (Selection)
Genomic prediction/selection can be traced to the use of marker-based kinship in the Best Linear Unbiased Prediction (BLUP) method, currently known as genomic BLUP or gBLUP (Bernardo 1994 ; Zhang et al. 2007 ; VanRaden 2008 ). The method uses all markers covering the whole genome to define the kinship among individuals to estimate their EBV. A different strategy is to estimate the effects of all markers and sum them together to predict individuals’ total genetic effects (Meuwissen et al. 2001 ). To avoid the overfitting problem in the fixed-effect model, these markers are fitted as random effects simultaneously. A variety of restrictions and assumptions are applied to these random effects and their prior distributions under the Bayesian theorem. Different methods were named according to different priors, such as Ridge Regression (no prior), Bayes A, B, Cpi, and LASSO. Different from GWAS, the best GS method is dataset dependent. For plogenetic traits, gBLUP performs well. For traits with low heritability, the compressed BLUP performs well (Wang et al. 2019 ). Enrichment of prediction methods (e.g. Bayesian alphabets, BLUP alphabets, and machine learning), and optimization of their usage on particular datasets (e.g. MMAP) are beneficial to improve prediction accuracy.