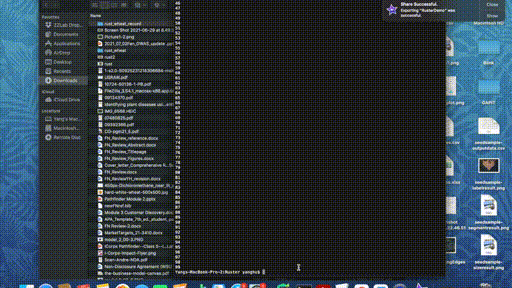
Stripe rust is one of the devastating diseases in wheat and barley with airborne urediniospores that cycle multiple times in one growing season causing large-scale epidemics and 100% yield loss. Applying fungicide at initial stages is the key to control the disease, which currently relies on inefficient human observations. Infections can not be easily detected until reaching high infection rates such as 1%, which is already too late. The solutions could be continuing the current practice of blindly applying fungicide early, or to developing methods capable of detecting at a lower rate. Based on images and videos from close-up drones and smartphones, we developed a neural network named StripeRustNet as a plugin of the ROOSTER software to process multiple images and report the incidences of stripe rust. StripeRustNet was trained on ResNet pre-trained with ImageNet. The training process includes using automatic labeling image tiles with ROOSTER and semi-automatic labeling with human corrections assisted by the predictions from StripeRustNet in an online learning fashion. Both cross-validations and independent validations across multiple conditions, such as species, locations, and farms, indicated that StripeRustNetl had 90% of detection power at only a 10% false discovery rate. A field with a 1/100,000 infection rate can be detected with 300 images, which took less than 10 minutes using smartphones or drones covering large areas, suggesting 100 times improvement of detection ability.